Make AI Cheap Again
By Dr. Steven Waterhouse – Guest Writer Updated April 18, 2025
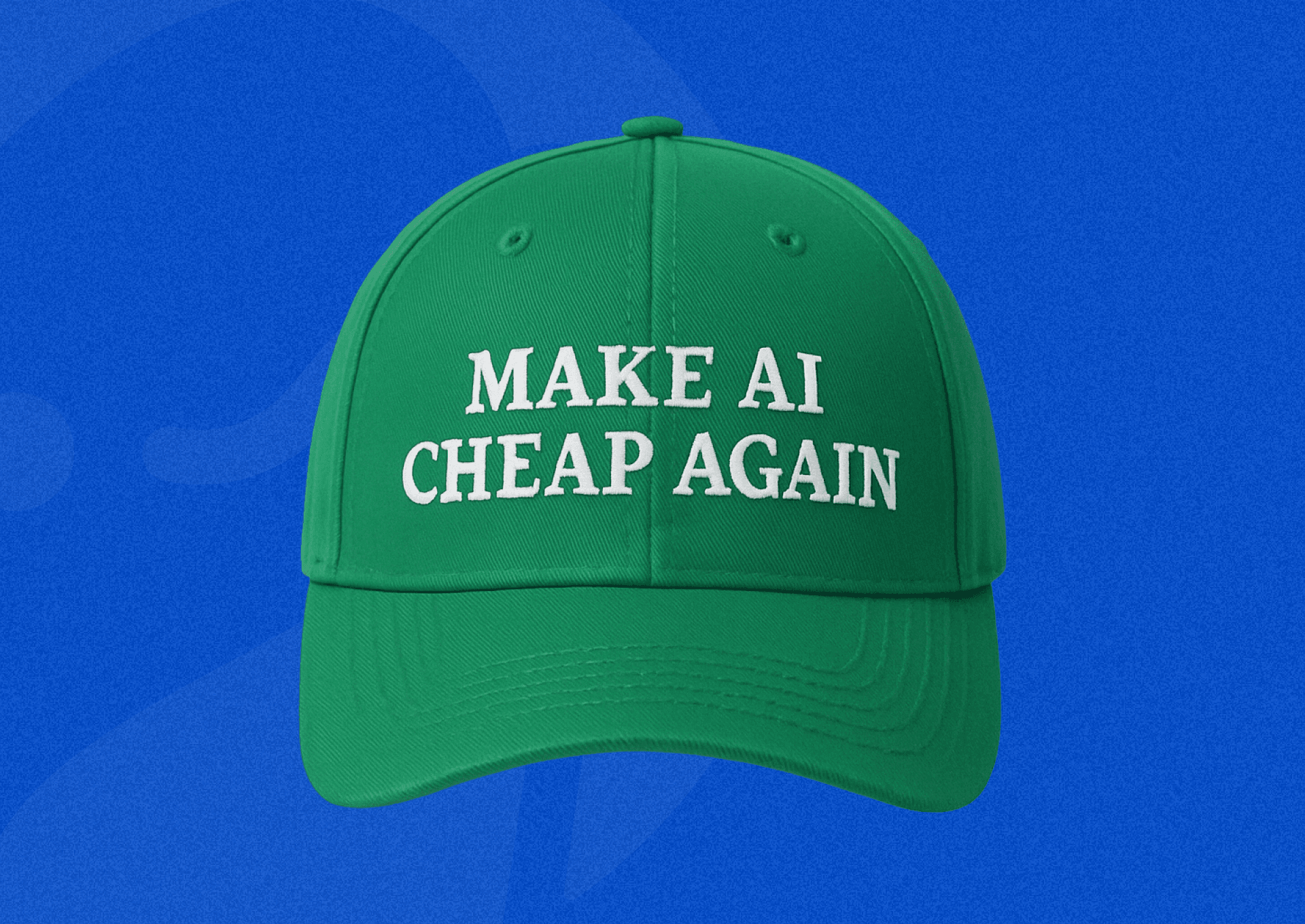
Summary
- AI companies are being forced to shift from a “scale at all costs” mindset to one obsessed with cost per token, energy consumption, and return on investment.
- Not everyone’s raised money. Not everyone has unlimited runway. MACHA is born in this context.
- Distributing computation across contributor networks significantly reduces costs, a necessity for the AI future we all dream of.
Why economic pressure ushers in a new era of distributed, efficient, and cost-aware AI systems.
A new wave of economic pressure is reshaping the AI calculus. A new US President in office with aggressive tariff agenda have triggered a rapid realignment in global trade. Supply chains are tightening, markets are correcting, and companies are feeling the pressure. Budgets across the board (including once-sacred AI budgets) are now being slashed, and leadership is demanding ROI.
While industry giants and hyperscalers will continue developing foundational models powered by mega clusters, alongside them a new movement is emerging. Scrappy, resourceful teams with a relentless focus on doing more with less. Welcome to the MACHA era: Make AI Cheap Again
Current Market Dynamics: More than a Correction
The current market downturn isn’t just a correction. It’s somewhere between a structural reset and a fundamental realignment. Rising tariffs on critical tech imports, including semiconductors and infrastructure hardware, are hitting hyperscalers and startups alike. AI companies are being forced to shift from a “scale at all costs” mindset to one obsessed with cost per token, energy consumption, and return on investment.
Financial scrutiny has intensified. Companies that previously approved AI initiatives with minimal oversight now demand concrete justifications. What's the point (and payback period) of that language model integration? Is there a more cost-effective alternative to that GPU cluster? Can we move faster? Can we build more cheaply? Can we accelerate development while reducing expenses?
Open Source & “Good Enough”: Doing More w/ Less
Sun Microsystems (where I worked at the turn of the century, ticker: $JAVA) offers a compelling parallel. In its heyday, Sun reigned supreme with high-performance servers and sleek Solaris boxes. But then came open-source: Linux, Apache, and rows of cheap, distributed x86 hardware. Ultimately, Sun couldn't compete.
Today's AI landscape is similar. The monolithic, capital-intensive approach dominated by hyperscalers and Nvidia-powered mega-clusters is increasingly unsustainable. Instead, an ecosystem is emerging of open-source tools, flexible compute frameworks, and alternative cloud providers delivering 90% of the performance at 10% of the cost.
In the MACHA world, "good enough" compute is a feature, not a flaw.
For companies, this shift is existential, not theoretical. Consumers don’t care if their AI assistant is centralized or decentralized. They care that it works. But founders, companies, and developers live inside the tradeoffs. They feel every constraint, every cloud invoice, every delay in model throughput. Not everyone’s raised money. Not everyone has unlimited runway. MACHA is born in this context. Born of necessity, not ideology. It's the genesis of a new AI (and societal) reality: do more with less.
The MACHA “Future Stack”
Making AI Cheap Again would only be possible with a robust toolkit. Thankfully a “future” stack exists, upon which the next wave of AI will get built.
- Distributed Architectures & Democratized Hardware: Forward-thinking developers are exploring alternatives to premium GPUs, incorporating consumer-grade hardware, ARM processors, RISC-V architectures, and application-specific integrated circuits into AI workflows.
- Open Source Frameworks: Tools like vLLM, MLC, and Hugging Face's ecosystem have dramatically lowered entry barriers while creating sustainable business models through enterprise support services.
- Low-Cost Cloud Alternatives: Specialized providers such as Core Weave, Vast AI, and Lambda API are challenging established players by offering substantial cost advantages for training and inference, including by unlocking idle compute through peer-to-peer marketplaces.
- Training and Inference Outside of Mega Clusters: Companies like Prime Intellect and Nous Research are enabling training and inference outside of traditional mega clusters using distributed GPUs and open-source models.
- Local Intelligence on the Edge: Apple's Neural Engine exemplifies a tiered intelligence model where sensitive, real-time tasks are processed locally on-device, while complex computations are selectively offloaded to the cloud. Enhances speed, efficiency, and privacy by keeping data close to the user and reserving cloud resources for only what’s necessary.
The Continuum of Compute
The future of computation is a continuum from massive GPU mega clusters to billions of distributed edge devices in homes, factories, and pockets worldwide. The development of software enabling AI-powered applications to be built and operated across this continuum is an enormous opportunity.
Distributing computation across contributor networks significantly reduces costs. When compute is coordinated, inference that once required a centralized cluster can now be distributed across idle devices or crowd-sourced infrastructure. These systems reduce overhead, expand geographic reach, and enable better pricing. Intelligence delivered peer-to-peer (or trained collaboratively across a mesh of participants) costs less and scales naturally with demand.
What’s left is a world where cost efficiency and resilience come bundled together. If the first wave of AI was about capability, the next will be about efficiency. The most promising opportunity today involves developing tools, infrastructure, and platforms that reduce the cost of delivering intelligence at scale.
Internet-Scale OS for AI
This is the Internet-Scale Operating System for AI: a distributed computing environment designed to operate across the compute continuum, from datacenter megacluster GPUs to local inference on phones, drones, and embedded sensors.
It’s horizontal and vertical. It’s scalable and ambient. It’s a dynamic matrix being built by teams who see the MACHA moment not just as a constraint but as a design brief.
Because the MACHA movement isn’t a Private Equity play. It isn’t just more data, more compute, nor is it simply ruthless austerity and cost cutting to juice returns. It’s about optimization, scale, and resilience. Intelligence shouldn’t be scarce, it should be abundant. If the future of AI is truly global and ambient, we need to reduce the costs of building, deploying, and maintaining these systems by orders of magnitude.
The End Game
The next transformative AI breakthrough won’t come from a trillion-dollar company. As we saw with DeepSeek, innovation can come from anywhere, at any time.
In fact, it might come from nothing more than a smart team, a cheap cluster, and a better economic model. Getting that team online means building an Internet-scale OS for AI that transforms the compute continuum into a unified intelligence fabric. MACHA.
Founder & CEO of Nazaré Ventures
Dr. Steven “Seven” Waterhouse is the Founder & GP of Nazaré Ventures, an early stage AI Venture Fund. He’s been active in building and investing in tech, Web3 and AI since 1997. Steven is a staunch defender of personal privacy as a basic right and is also an avid waterman who loves the cold waters of the Pacific and Atlantic west coasts.
Join the Beluga Brief
Dive deep into weekly insights, analysis, and strategies tailored to you, empowering you to navigate the volatile crypto markets with confidence.
Never be the last to know
and follow us on X